Keeeper's Results
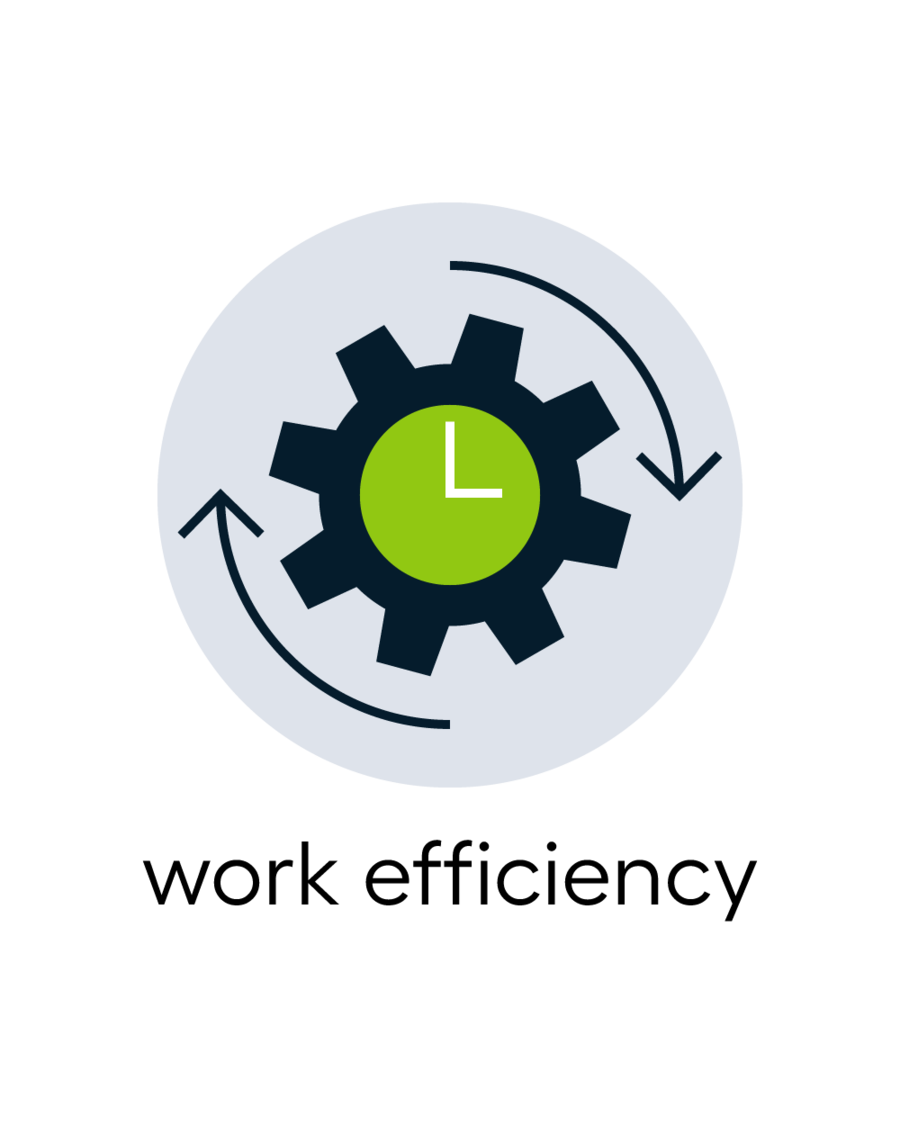
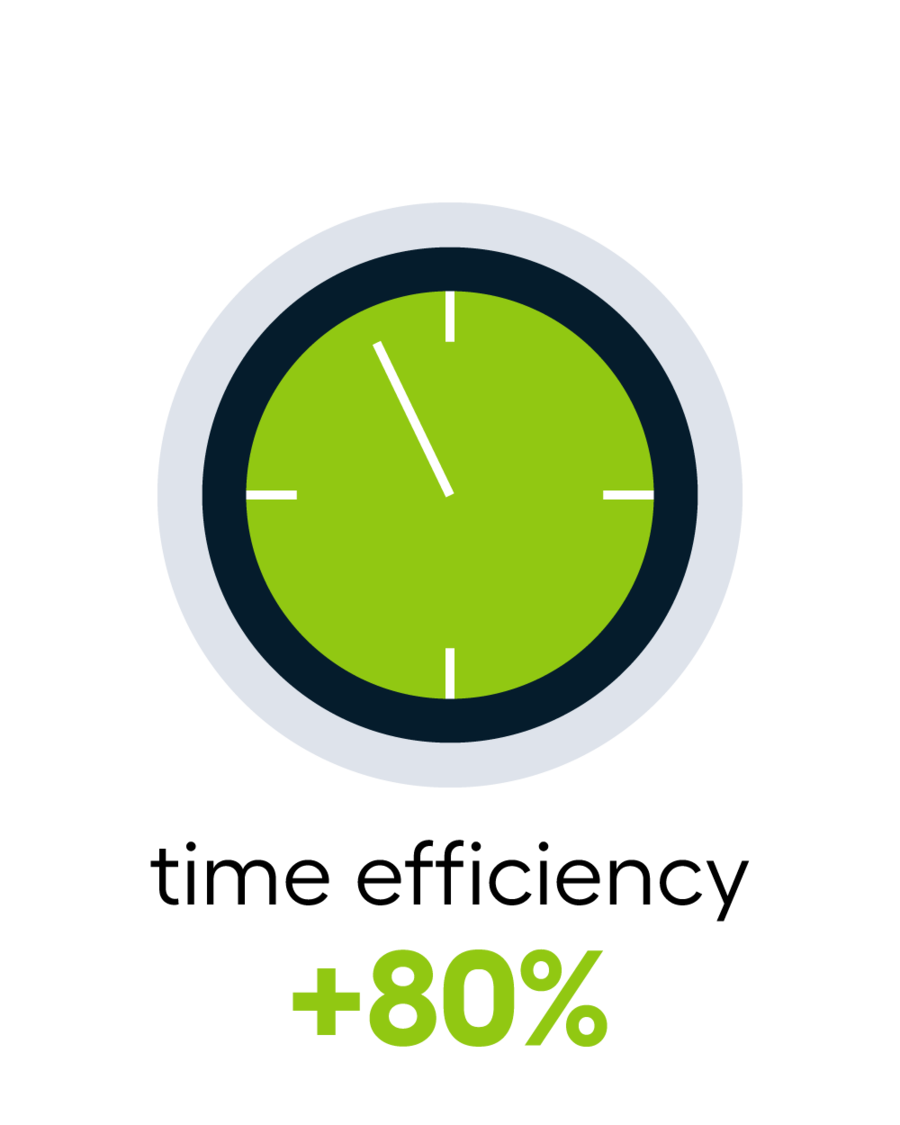
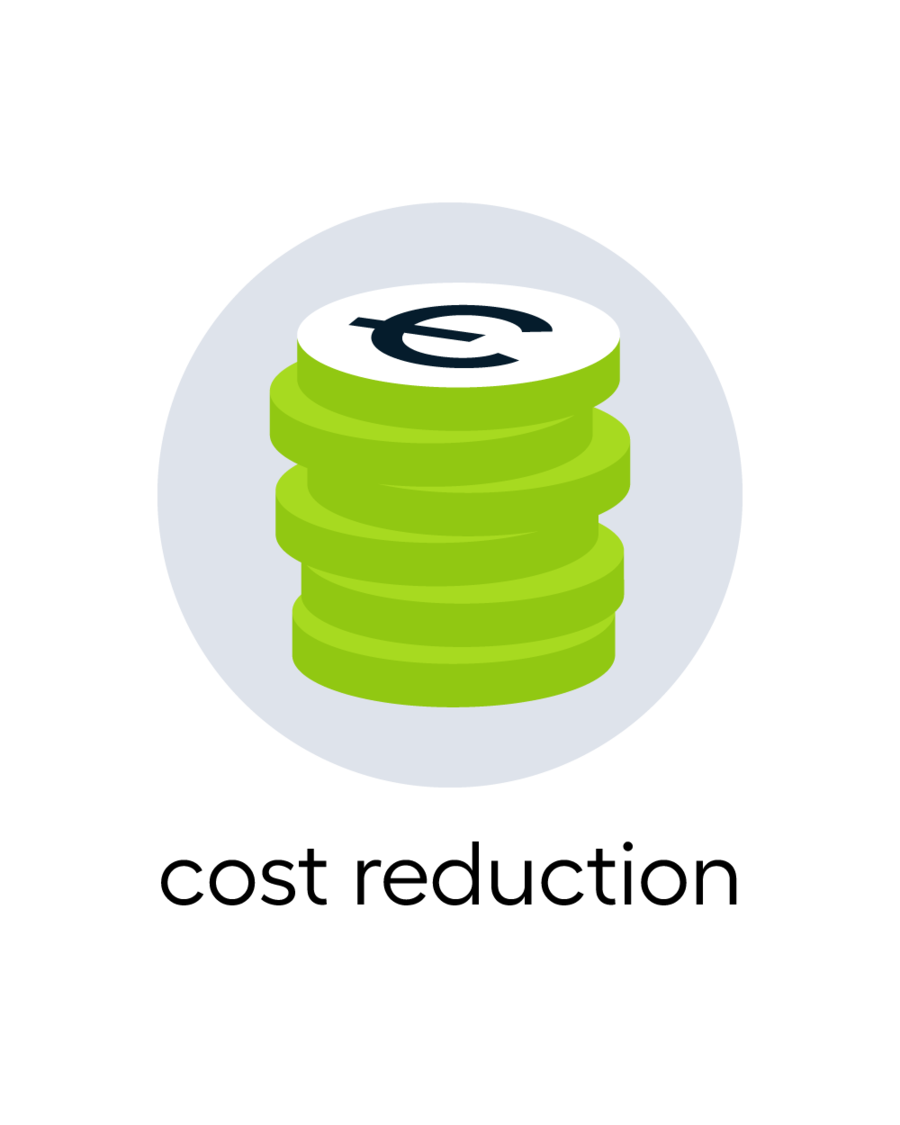
About Keeeper
Keeper specializes in the development, design and manufacture of plastic household products for daily use. The focus is on creating sustainable products with a functional and well-thought-out design.
Challenge
At Keeper they have more than 50 injection molding machines, and efficiently planning these machines is a significant challenge. There are several requirements to take into account, making the planning process more complex. An example of this is that the plastic color must be planned from light to dark, otherwise it does not meet the quality requirements. In addition, the capabilities and limitations of each machine must be considered, such as the changeover time between two productions. It must also be determined which mold is suitable for which machine. A mold can fit on multiple machines, but can of course only be used once at a time.
Until recently, the planning process was performed manually using data from SAP, resulting in inaccurate and time-consuming planning. In other words, there was room for significant improvement.
Solution
In a collaboration between Keeper and Nimble, several automated (RPA) bots have been developed, all with one common goal: planning production orders. The first bot is responsible for data preparation, including filtering, cleaning, and predicting. We use a Neural Network for predictions, which allows us to predict the lead times of specific productions. This predicted data is ultimately used to feed the main bot with data.
The second bot, also called the main bot, performs the most complex task: creating an optimized schedule based on the data from the first bot and the information from SAP. The SAP data contains the orders that need to be produced. This bot uses artificial intelligence (AI) to search through thousands of combinations to generate optimal planning, with the aim of efficiently deploying all machines, planning orders and much more. The quality of the planning is of the utmost importance. This bot can create a schedule for more than 50 different machines with more than 5,000 orders within 5 minutes, taking into account all relevant parameters such as limitations and availability. The emphasis of the planning can vary depending on the objective, whether it concerns quality (colour change), machine utilization or speed.
The last bot is optional and uses supervised learning. A human planner has the ability to retrospectively revise the schedule in the AI's graphical user interface (GUI), for example by adjusting the priority of the schedule due to changes in demand or unexpected events. After implementing changes, this bot recalculates all relevant variables and communicates this both with the planner to clarify the impact of the choices, and with the main bot so that it can continue to learn.
Result
Thanks to the use of these bots, more than 80% of the production order planning process at Keeper can now be carried out automatically. This gives the opportunity to free up time for other tasks instead of time-consuming schedules. Moreover, the accuracy of planning has never been higher, and it is also available within minutes. The bots will continue to improve in the future with new data, and by adjusting variables we can determine which aspects are most important.